Confidence interval
A confidence interval, or CI, (also called confidence interval, confidence interval, or expected range) is, in statistics, an interval intended to indicate the precision of the lag estimate of a parameter (e.g., a mean). The confidence interval indicates the range that includes with a certain probability (the confidence level) the parameter of a distribution of a random variable.
If a random experiment is repeated many times in an identical manner, the frequently chosen 95% confidence interval will contain the fixed, unknown, "true" parameter 95% of the time.
The frequently encountered formulation that the true value lies with 95 % probability in the currently available confidence interval is, strictly speaking, incorrect, since the true value is fixed and does not behave stochastically. The correct formulation would be: the true value lies for 95 % of all samples in the respective calculated confidence interval, because the upper and lower limits of the confidence interval depend on the present sample and are therefore random variables, i.e. stochastic. An alternative correct formulation is: when calculating a confidence interval, its interval boundaries enclose the true parameter 95% of the time and not 5% of the time. The confidence interval is constructed such that the true parameter β is covered with probability when the estimation procedure is repeated for many samples, where α
the so-called level of error.
Estimating parameters using confidence intervals is called interval estimation, and the corresponding estimator is called a range or interval estimator. One advantage over point estimators is that a confidence interval can be used to read significance directly: a wide interval for a given confidence level indicates a small sample size or strong variability in the population.
Confidence intervals are distinguished from forecast intervals and confidence and forecast bands.
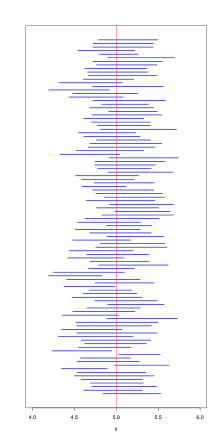

Confidence intervals at the 95% level for 100 samples of size 30 from a normally distributed population. Of these, 94 intervals cover the exact expected value μ = 5; the remaining 6 do not.
Definition
For a fixed given γ, a γ
-confidence interval for
to the confidence level γ
(also: a γ
-confidence interval) by the two - based on a random sample
- based on a random sample
and
which are
satisfy. The statistics and
are the bounds of the confidence interval, for which always
assumed. The confidence level γ
is also called the coverage probability. The realizations
and
of and
respectively,
form the estimation interval
. The bounds of the confidence interval are functions of the random sample
and therefore also random. In contrast, the unknown parameter is
fixed. Repeating the random experiment in an identical way, a γ -confidence interval
will cover the unknown parameter
in γ
all cases. However, since the unknown parameter
is not a random variable, one cannot say that
lies in a γ
-confidence interval with probability γ Such an interpretation is reserved for the Bayesian counterpart of confidence interval, called credibility intervals. The confidence level γ
is also called the coverage probability. Often one sets γ
. The probability
can be interpreted as a relative frequency: If one uses intervals for a large number of confidence estimates, each of which
has the level the relative frequency with which the concrete intervals cover the parameter approaches the value
.
Formal definition
General conditions
Given a statistical model and a function
,
which is also called parameter function in the parametric case. The set Γ contains the values that can be the result of an estimation. Usually, Γ
Confidence interval
An illustration
is called a confidence interval, confidence region, range estimator, or range estimator if it satisfies the following condition:
- For all γ
, the set
contained in (M)
Thus, a confidence region is a mapping that assigns to each observation an
initially arbitrary subset of Γ (
is here the power set of the set Γ
, that is, the set of all subsets of Γ
)
Condition (M) ensures that all sets can be assigned a probability. This is needed to define the confidence level.
Confidence interval
If Γ and if
always an interval for any , then
also called a confidence interval.
If confidence intervals are defined in the form
,
is defined, then also called the upper confidence bound and
the lower confidence bound.
Confidence level and level of error
Given a confidence region . Then
is called a confidence region at the confidence level or certainty level
if
.
The value α is then also called the level of error. A more general formulation is possible with shape hypotheses (see Shape Hypotheses#Confidence Ranges for Shape Hypotheses).
For the above-mentioned special cases with confidence intervals with upper and lower confidence limits, the following is thus obtained
respectively
and
Questions and Answers
Q: What is a confidence interval in statistics?
A: A confidence interval is a special interval used to estimate a parameter, such as the population mean, giving a range of acceptable values for the parameter instead of a single value.
Q: Why is a confidence interval used instead of a single value?
A: A confidence interval is used instead of a single value to account for the uncertainty of estimating a parameter based on a sample, and to give a likelihood that the real value of the parameter is within the interval.
Q: What is a confidence level?
A: A confidence level is the likelihood that the parameter being estimated is within the confidence interval, and is often given as a percentage (e.g. 95% confidence interval).
Q: What are confidence limits?
A: Confidence limits are the end points of a confidence interval, which define the range of acceptable values for the parameter being estimated.
Q: How does the confidence level affect the confidence interval?
A: In a given estimation procedure, the higher the confidence level, the wider the confidence interval will be.
Q: What assumptions are required to calculate a confidence interval?
A: The calculation of a confidence interval generally requires assumptions about the nature of the estimation process, such as the assumption that the distribution of the population from which the sample came is normal.
Q: Are confidence intervals robust statistics?
A: Confidence intervals, as discussed below, are not robust statistics, though adjustments can be made to add robustness.