Chi-squared distribution
The chi-square distribution or χ distribution (older name: Helmert-Pearson distribution, after Friedrich Robert Helmert and Karl Pearson) is a continuous probability distribution over the set of non-negative real numbers. Usually, "chi-squared distribution" means the central chi-squared distribution. The chi-squared distribution has a single parameter, namely the number of degrees of freedom
.
It is one of the distributions that can be derived from the normal distribution Given
random variables
that are independent and standard normally distributed, then the chi-squared distribution with
degrees of freedom is defined as the distribution of the sum of squared random variables
. Such sums of squared random variables occur in estimators such as sampling variance for estimating empirical variance. Thus, among other things, the chi-squared distribution allows a judgment to be made about the compatibility of a presumed functional relationship (dependence on time, temperature, pressure, etc.) with empirically determined measurement points. For example, can a straight line explain the data, or do we need a parabola or perhaps a logarithm? One chooses different models, and the one with the best goodness of fit, the smallest chi-square value, provides the best explanation of the data. Thus, by quantifying the random fluctuations, the chi-square distribution puts the choice of different explanatory models on a numerical basis. Moreover, once one has determined the empirical variance, it allows one to estimate the confidence interval that includes the (unknown) value of the variance of the population with some probability. These and other applications are described below and in the article Chi-Square Test.
The chi-squared distribution was introduced in 1876 by Friedrich Robert Helmert, the name comes from Karl Pearson (1900).
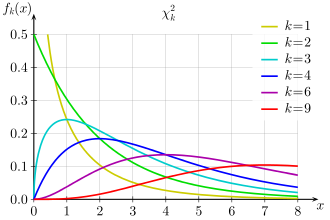

Densities of the chi-squared distribution with different number of degrees of freedom k
Definition
The square of a standard normally distributed random variable follows a chi-squared distribution with one degree of freedom:
.
Furthermore, if are jointly stochastically independent chi-squared distributed random variables, then their sum is chi-squared distributed with the sum of the respective degrees of freedom
.
Thus, the chi-squared distribution is reproducible. Let be stochastically independent and standard-normally distributed random variables, then their sum-of-squares
that it is chi-squared distributed with the number of degrees of freedom
:
.
The character is a shorthand notation for "follows the distribution". For example,
; also often written as
The random variable
follows a chi-squared distribution with number of degrees of freedom
. The sum of squared quantities cannot take negative values.
In contrast, for the simple sum with distribution symmetric about the zero point.
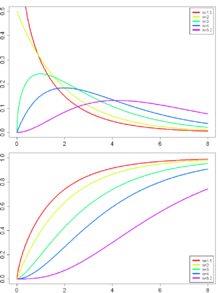

Density and distribution of multiple chi-square distributed random variables.
Density
The density of the χ
distribution with
degrees of freedom has the form:
Here Γ stands for the gamma function. The values of Γ
can be calculated with
.
Calculate
Distribution function
The distribution function can be written using the regularized incomplete gamma function:
If is a natural number, then the distribution function can be represented (more or less) elementarily:
where denotes the error function. The distribution function describes the probability that χ
lies in the interval
Properties
Expected value
The expected value of the chi-square distribution with degrees of freedom is equal to the number of degrees of freedom
.
Thus, assuming a standard normally distributed population, if the variance of the population is correctly estimated, the value χ should be close to 1.
Variance
The variance of the chi-square distribution with degrees of freedom is equal to 2 times the number of degrees of freedom
.
Mode
The mode of the chi-squared distribution with degrees of freedom is
for
.
Skew
The skewness γ of the chi-squared distribution with
degrees of freedom is
.
The chi-square distribution has a positive skewness, i.e., it is left-skewed or right-skewed. The higher the number of degrees of freedom the less skewed the distribution is.
Kurtosis
The kurtosis (kurtosis) β of the chi-squared distribution with
degrees of freedom is given by
.
The excess γ over the normal distribution thus results in γ
. Therefore, the higher the number of degrees of freedom
, the lower the excess.
Moment generating function
The moment generating function for has the form
.
Characteristic function
The characteristic function for from the moment generating function as:
.
Entropy
The entropy of the chi-squared distribution (expressed in nats) is
where ψ(p) denotes the digamma function.
Non-Central Chi-Square Distribution
If the normally distributed random variables are not centered with respect to their expected value μ if not all μ
), the noncentral chi-squared distribution is obtained. It has as a second parameter besides
the noncentrality parameter λ
Let , then
with λ
.
In particular, it follows from and
that
is
A second way to generate a non-central chi-squared distribution is as a mixture distribution of the central chi-squared distribution. Here is
,
if is drawn from a Poisson distribution.
Density function
The density function of the non-central chi-squared distribution is
for
,
for
The sum over j leads to a modified Bessel function of first genus . This gives the density function the following form:
for
.
Expected value and variance of the noncentral chi-squared distribution and
into the corresponding expressions of the central chi-squared distribution, as does the density itself when λ
Distribution function
The distribution function of the noncentral chi-squared distribution can be expressed using the Marcum function Q
Example
Take measurements of a quantity
, which come from a normally distributed population. Let be
the empirical mean of the
measured values and
the corrected sample variance. Then, for example, the 95% confidence interval for the variance of the population σ specified:
where χ by
and χ
by
, and therefore also χ
. The limits follow from the fact that
is distributed as χ
Derivation of the distribution of the sample variance
Let a sample of
measurements, drawn from a normally distributed random variable
with empirical mean
and sample variance
as estimators for expected value μ
and variance σ
the population.
Then it can be shown, that is distributed as χ
.
To do this, Helmert transforms the into new variables
using an orthonormal linear combination. The transformation is:
The new independent variables are
normally distributed like with equal variance σ
, but with expected value
both due to the convolution invariance of the normal distribution.
Moreover, for the coefficients in
(if
,
) because of orthonormality
(Kronecker delta) and thus
Therefore, the sum of the squares of the deviations is now given by
and finally after division by σ
The expression on the left hand side is apparently distributed like a sum of squared standard normally distributed independent variables with summands, as required for χ
Thus, the sum is chi-squared distributed with degrees of freedom
, while by definition of the chi-squared sum
. One degree of freedom is "consumed" here, because due to the centroid property of the empirical mean
the last variation is
already
determined by the first Consequently, only
variances vary freely and one therefore averages the empirical variance by
dividing by the number of degrees of freedom
Relationship to other distributions
Relationship to gamma distribution
The chi-squared distribution is a special case of the gamma distribution. If , then
Relationship to normal distribution
- Let
be independent and standard normally distributed random variables, then their sum of squares
that it is chi-squared distributed with the number of degrees of freedom
:
.
- For
, is
approximately standard normally distributed.
- For
the random variable X n {\displaystyle
normally distributed, with expected value n {\displaystyle n}
standard deviation 2 n {\displaystyle for a noncentral chi-squared distribution with expected value
and standard deviation
.
Relationship to exponential distribution
A chi-squared distribution with 2 degrees of freedom is an exponential distribution with parameter λ
.
Relationship to Erlang distribution
A chi-squared distribution with degrees of freedom is identical to an Erlang distribution
with
degrees of freedom and λ
.
Relationship to F-Distribution
Let χ and χ be
independent chi-squared distributed random variables with
and
degrees of freedom, then the quotient is
F-distributed with numerator degrees of freedom and
denominator degrees of freedom.
Relationship to Poisson distribution
The distribution functions of the Poisson distribution and the chi-square distribution are related in the following way:
The probability of finding or more events in an interval within which one expects on average λ
events is like the probability that the value of χ
Namely, it holds
,
with and
as regularized gamma functions.
Relationship to continuous uniform distribution
For even one can form the χ
-distribution as an
-fold convolution using the uniformly continuous density
:
,
where the are independent uniformly continuously distributed random variables.
For odd , however, the following applies
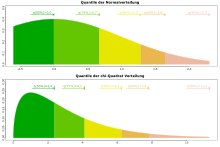

Quantiles of a normal distribution and a chi-square distribution
Derivation of the density function
The density of the random variable χ , with
independent and standard normally distributed, is given by the joint density of the random variables
. This joint density is the
-fold product of the standard normal distribution density:
For the density we are looking for:
where
In the limit, the sum in the argument of the exponential function is equal to . It can be shown that the integrand can be drawn as
before the integral and the limit.
The remaining integral
corresponds to the volume of the shell between the sphere with radius and the sphere with radius
,
where gives the volume of the n-dimensional sphere with radius R.
It follows:
and after substituting into the expression for the density we are looking for:
Quantile function
The quantile function the chi-squared distribution is the solution of the equation
and thus in principle can be calculated via the inverse function. Concretely applies here
with as inverse of the regularized incomplete gamma function. This value
is
entered in the quantile table under the coordinates
and
Quantile function for small sample size
For a few values (1, 2, 4) one can also specify the quantile function alternatively:
where denotes the error function,
denotes the lower branch of the Lambertian W-function, and
denotes the Euler number.
Approximation of the quantile function for fixed probabilities
For certain fixed probabilities the associated quantiles
obtained by the simple sample size function
with parameters from the table, where
denotes the signum function, which simply represents the sign of its argument:
| 0,005 | 0,01 | 0,025 | 0,05 | 0,1 | 0,5 | 0,9 | 0,95 | 0,975 | 0,99 | 0,995 |
| −3,643 | −3,298 | −2,787 | −2,34 | −1,83 | 0 | 1,82 | 2,34 | 2,78 | 3,29 | 3,63 |
| 1,8947 | 1,327 | 0,6 | 0,082 | −0,348 | −0,67 | −0,58 | −0,15 | 0,43 | 1,3 | 2 |
| −2,14 | −1,46 | −0,69 | −0,24 | 0 | 0,104 | −0,34 | −0,4 | −0,4 | −0,3 | 0 |
Comparison with a χ table shows
relative error below 0.4% for and n > 10
Since the χ
-distribution for large
transitions to a normal distribution with standard deviation
, the parameter
from the table, which has been freely adjusted here, at the corresponding probability
approximately the size of
times the quantile of the normal distribution (
), where
denotes the inverse of the error function.
The 95% confidence interval for the variance of the population from the Example section can be obtained, e.g. with the two functions from the lines with
and
plotted in a simple way as a function of
.
The median is located in the column of the table with .